Advancing Pharmacovigilance with ML: Unlocking the Potential of Data-driven Safety Surveillance
- sales293608
- 23 thg 7, 2023
- 3 phút đọc
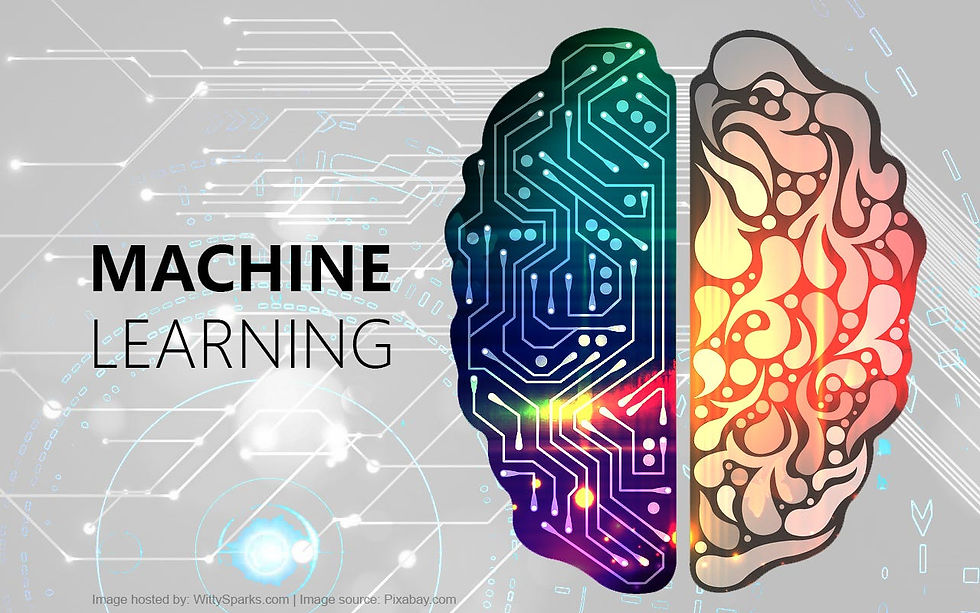
Introduction:
Pharmacovigilance, the science of monitoring and evaluating the safety of medical products, is vital in ensuring patient well-being. Traditionally, pharmacovigilance relied on manual reporting and data analysis, which could be time-consuming and might not capture all safety signals. However, with the rapid advancements in Machine Learning (ML) and Artificial Intelligence (AI), a new era of data-driven safety surveillance has emerged. In this blog, we explore how Machine Learning is revolutionizing pharmacovigilance, enabling early detection of adverse events, improving signal detection, and empowering healthcare professionals to make informed decisions for better patient outcomes.
1. Early Detection of Adverse Events: Machine Learning algorithms excel at analyzing vast amounts of data quickly and efficiently. In pharmacovigilance, this capability is critical for early detection of adverse events associated with specific drugs. By continuously analyzing electronic health records, social media data, and other real-world evidence, ML algorithms can identify potential safety signals in near real-time, allowing healthcare professionals to take prompt actions to protect patients.
2. Signal Detection and Analysis: Pharmacovigilance databases are continually growing with new safety information. Machine Learning models can effectively analyze this data, identifying patterns and trends that may not be evident through manual review. ML algorithms can pinpoint potential associations between drugs and adverse events, helping pharmacovigilance teams prioritize their investigations and allocate resources efficiently.
3. Automated Adverse Event Reporting: Manual adverse event reporting can be labor-intensive and prone to errors. Machine Learning offers the potential to automate the reporting process, extracting relevant information from unstructured data sources such as medical narratives, case reports, and drug labels. This automation streamlines the adverse event reporting process, allowing pharmacovigilance professionals to focus on data analysis and decision-making.
4. Pharmacovigilance in Real-Time: The traditional pharmacovigilance process often involves retrospective analysis of safety data. Machine Learning enables real-time surveillance, facilitating proactive identification of safety signals and timely intervention. This real-time approach enhances the ability to monitor drug safety continuously and ensures a more responsive and patient-centric healthcare system.
5. Personalized Safety Profiles: Machine Learning's ability to process vast amounts of patient data enables the creation of personalized safety profiles. By considering individual patient characteristics, such as genetic factors, medical history, and concomitant medications, ML algorithms can predict an individual's risk of experiencing adverse drug reactions. This personalized approach allows healthcare providers to tailor treatment plans and minimize adverse events, ultimately improving patient safety and treatment outcomes.
6. Data Quality and Bias Mitigation: Machine Learning models are only as reliable as the data they are trained on. Ensuring high data quality is essential in pharmacovigilance to avoid biased or erroneous conclusions. Researchers and data scientists are working diligently to develop algorithms that can detect and correct for potential biases in the data, making ML-driven pharmacovigilance more accurate and robust.
7. Drug Repurposing and Pharmacogenomics: Machine Learning has enabled drug repurposing by analyzing existing drug databases to identify potential new therapeutic uses for existing medications. Additionally, pharmacogenomics, which studies the interaction between genetics and drug response, is benefitting from ML applications to predict individual patient responses to specific drugs, guiding personalized treatment plans and minimizing adverse reactions.
Conclusion:
Machine Learning is rapidly transforming the field of pharmacovigilance, revolutionizing how adverse events are detected, analyzed, and reported. The ability of ML algorithms to process vast amounts of data in real-time enables early identification of safety signals, paving the way for proactive and patient-centric healthcare practices. As Machine Learning continues to advance and data quality improves, pharmacovigilance will become more accurate, efficient, and personalized, ultimately enhancing patient safety and contributing to better public health outcomes. Embracing the power of Machine Learning in pharmacovigilance is not just a technological leap, but a significant stride towards a safer and more effective healthcare ecosystem.
Comments